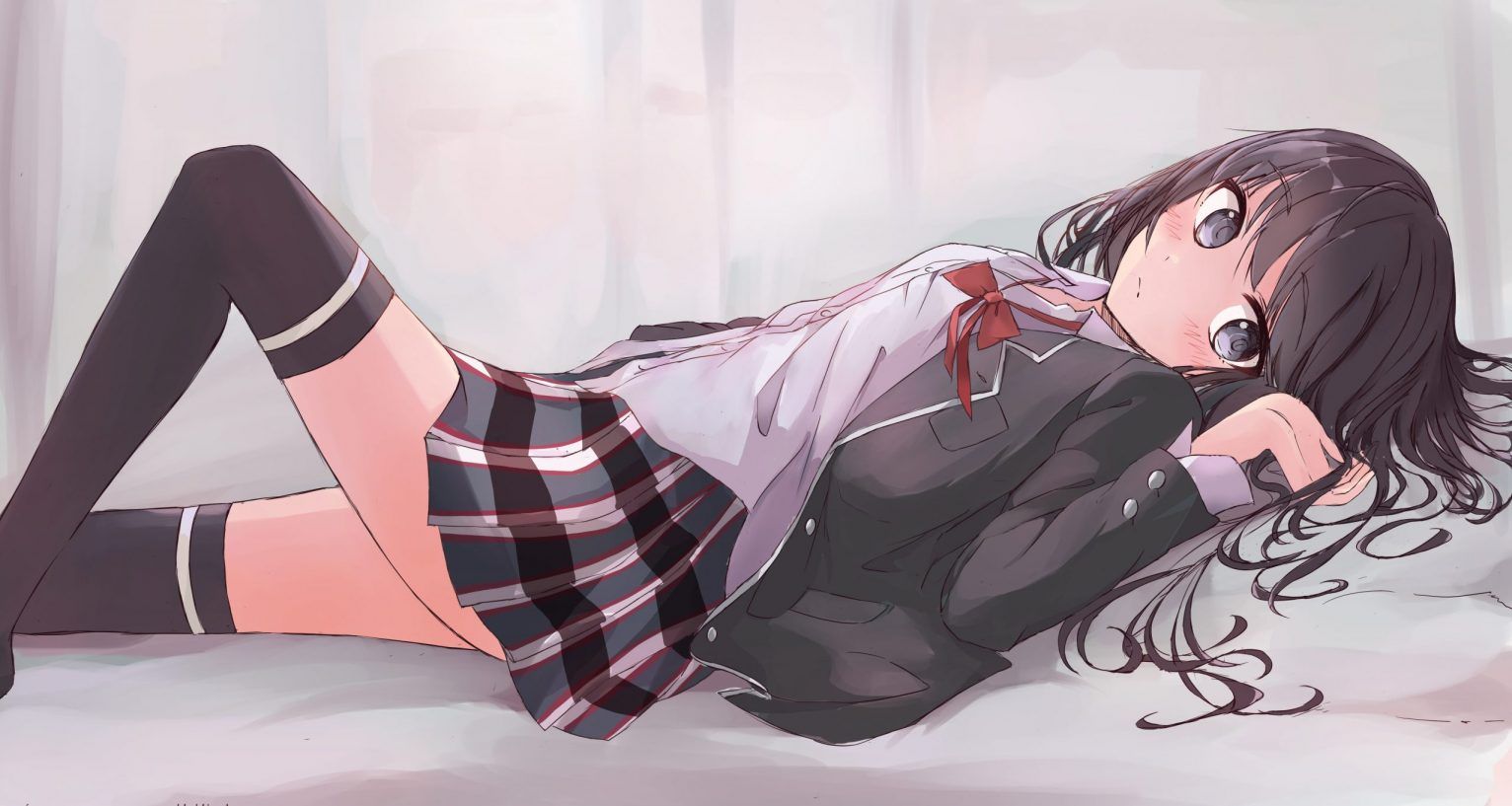
概率论与数理统计笔记(3)——联合分布
Chapter 3:联合分布
1、随机向量
定义
(X1,⋯,Xn)
称为(n 维)随机向量,任意 Xi 是随机变量。
(联合)累积分布函数(cdf)
F(x1,⋯,xn)=P(X1≤x1,⋯,Xn≤xn)∀(x1,⋯,xn)∈Rn
注:n=2 时为二元分布,常用 (X,Y) 和 F(x,y) 表示。
2、离散分布
定义
任意 Xi 都是离散型,则称 (X1,⋯,Xn) 为离散型。
概率质量函数(pmf)
f(x1,⋯,xn)△=P(X1=x1,⋯,Xn=xn)∀(x1,⋯,xn)∈Rnf≥0,∑f(x1,⋯,xn)≡1
e.g. 多项分布。
3、连续分布
定义
若存在 f(x1,⋯,xn)≥0 使得 ∀I⊆Rn 可测集都有:
P((X1,⋯,Xn)∈I)=∫If(x1,⋯,xn)dx1⋯dxn
则称 (X1,⋯,Xn) 为连续型随机向量,f 为 X1,⋯,Xn 的概率密度函数(pdf)。
注:
1. pdf 积分恒为 1:
∫Rnf≡1
2. cdf 为 pdf 积分:
F(a,b)=∫b−∞∫a−∞f(x,y)dxdy
实例
e.g. 均匀分布(矩形)。
f(x,y)={1(b–a)(d–c)a<x<b,c<y<d0otherwisee.g. 二元正态分布。
(X,Y)∼N(μ1,μ2,σ21,σ22,ρ)f(x,y)=12πσ1σ21√1−ρ2exp{−12(1−ρ2)[(x−μ1σ1)2−2ρx−μ1σ1y−μ2σ2+(y−μ2σ2)2]}
大括号内为二次型。
{...}=−12→XTW→X→X=(x−μ1σ1y−μ2σ2),W=11−ρ2(1−ρ−ρ1)=ATAA=1√1−ρ2(1−ρ0±√1−ρ2)或1√1−ρ2(−1ρ0±√1−ρ2)
4、边际分布
定义
Fi(x)△=P(Xi≤x)=P(Xi≤x,−∞<Xj<+∞(∀i≠j))称其为边际 cdf。当 n=2 时,对于 (X,Y): FX(x)=P(X≤x)=limy→+∞F(x,y)FY(y)=P(Y≤Y)=limx→+∞F(x,y) e.g. P(X>a,Y>b)=1–FX(a)–FY(b)+F(a,b)
离散型(n=2)
P(X=x)=∑yP(X=x,Y=y)
e.g. (X,Y)——两次掷骰子点数。
P(X=1)=16,P(X≤2)=13
连续型(n=2)
FX(x)△=P(X≤x)=∫x−∞(∫∞−∞f(x,y)dy)dx
边际 pdf:
fX(x)=∫∞−∞f(x,y)dy
e.g. (X,Y)∼N(μ1,μ2,σ21,σ22,ρ)
fX(x)=∫∞−∞f(x,y)dy=1√2πσ1e−(x–μ1)22σ21,∀x∈R⇒X∼N(μ1,σ21)
同理可得:Y∼N(μ2,σ22)。
注:联合分布确定边际分布,反之则不对!
5、条件分布
以 n=2 为例。
离散型
P(X=ai,Y=bj)=Pij≥0 (i,j=1,2,⋯)P(X=ai|Y=bj)=P(X=ai,Y=bj)P(Y=bj)=Pij∑lPlj
需要 P(Y=bj)>0。
注:
1. ∑iP(X=ai|Y=bj)≡1。
2. 高维情况类似。
连续型
(X,Y) 的 pdf 为 f(x,y)。
P(X≤x|y≤Y≤y+dy)=P(X≤x,y≤Y≤y+dy)P(y≤Y≤y+dy)=∫x−∞∫y+dyyf(t,s)dsdt∫y+dyyfY(s)dsfX(x|y≤Y≤y+dy)=∫y+dyyf(x,s)ds∫y+dyyfY(s)ds→f(x,y)fY(y)(dy→0)
条件密度函数:
fX(x|y)△=f(x,y)fY(y)
需要 fY(y)>0。
fY(y|x) 类似定义。
注:f(x,y)=fX(x|y)fY(y)=fY(y|x)fX(x)
⇒fX(x)=∫∞−∞f(x,y)dy=∫∞−∞fX(x|y)fY(y)dy
——全概率公式。
fX(x|y)=f(x,y)∫∞−∞f(x,y)dx
⇒ Bayes 公式。
FX(a|y)=P(X≤a|Y=y)=∫a−∞fX(x|y)dx(P(Y=y)≡0)
多维情况类似。
e.g. (X,Y)∼N(μ1,μ2,σ21,σ22,ρ)
fY(y|x)=f(x,y)fX(x)=1√2πσ21√1–ρ2exp(−(y–(μ2+ρσ2σ1(x–μ1)))22(1–ρ2)σ22)
⇒ 给定 X=x 条件下 Y 的分布为 N(μ2+ρσ2σ1(x–μ1),(1–ρ2)σ22)。
中心为 μ2+ρσ2σ1(x–μ1)。
6、独立性
定义
(X,Y) 的 cdf 为 F(x,y),若 F(x,y)=FX(x)FY(y),∀x,y∈R,则称 X,Y 相互独立。
注:X,Y 独立 ⇔f(x,y)=fX(x)fY(y),∀x,y∈R。
连续型时为 pdf,离散型时为 pmf。
定义(高维推广)
设 (X1,⋯,Xn) 的 cdf 为 F(x1,⋯,xn),若 F(x1,⋯,xn)=F1(x1)⋅⋯⋅Fn(xn),∀x1,⋯,xn∈R,则称 X1,⋯,Xn 相互独立。
这里的 Fi(Xi) 为边际 cdf。
注:X1,⋯,Xn 独立 ⇔f(x1,⋯,xn)=f1(x1)⋅⋯⋅fn(xn),∀x1,⋯,xn∈R。
连续型时为 pdf,离散型时为 pmf。
定理
- 若 f(x1,⋯,xn)=g1(x1)⋅⋯⋅gn(xn),∀x1,⋯,xn∈R,则连续型随机变量 X1,⋯,Xn 独立,且 fi(xi) 与 gi(xi) 只差常数因子。
- 若 X1,⋯,Xn 独立,则 Y1=g1(X1,⋯,Xm),Y2=g2(Xm+1,⋯,Xn) 相互独立。
7、多个随机变量的函数
Y=g(X1,⋯,Xn)
直接法
e.g. Xi∼B(ni,p)(i=1,2) 相互独立,Y=X1+X2:
P(Y=k)=k∑k1=0P(X1=k1,X2=k–k1) (k=0,1,⋯,n1+n2)=k∑k1=0P(X1=k1)P(X2=k–k1)=k∑k1=0(n1k1)pk1(1–p)n1–k1(n2k–k1)pk–k1(1–p)n2–(k–k1)=[k∑k1=0(n1k1)(n2k–k1)]pk(1–p)n1+n2–k=(n1+n2k)pk(1–p)n1+n2–k⇒Y∼B(n1+n2,p)
注:对于 k1 无法取遍 0∼k 时,也有相同结果,此处略去讨论。
e.g. (X1,X2) 的 pdf 为 f(x1,x2),X1>0,Y=X2X1:
∀y,P(Y≤y)=P(X2X1≤y)=P(X2≤yX1)=∬
注:若 X_1, X_2 独立,则:
l(y) = \int_0^\infty x_1 f_1(x_1) f_2(x_1 y) \mathrm{d} x_1
密度函数变换法
(X_1, X_2) 的 pdf 为 f(x_1, x_2)。
\begin{cases} Y_1 = g_1(X_1, X_2) \\ Y_2 = g_2(X_1, X_2) \end{cases} \quad \quad \begin{cases} X_1 = h_1(Y_1, Y_2) \\ X_2 = h_2(Y_1, Y_2) \end{cases}
左侧映射可逆可微。
P \left ( (Y_1, Y_2) \in A \right ) \\ = P \left ( (X_1, X_2) \in B \right ) \\ = \int_B f(x_1, x_2) \, \mathrm{d} x_1 \mathrm{d} x_2 \\ = \int_A f(h_1(y_1, y_2), h_2(y_1, y_2)) |J| \, \mathrm{d} y_1 \mathrm{d} y_2 \\ \Rightarrow (Y_1, Y_2) \text{ 的 } pdf \text{ 为 } f(h_1(y_1, y_2), h_2(y_1, y_2)) |J|
其中 J 为雅克比行列式:
J = \begin{vmatrix} \dfrac{\partial h_1}{\partial y_1} & \dfrac{\partial h_1}{\partial y_2} \\ \dfrac{\partial h_2}{\partial y_1} & \dfrac{\partial h_2}{\partial y_2} \end{vmatrix}
e.g. X_1, X_2 的 pdf 为 f(x_1, x_2),Y = X_1 + X_2。
令 Z = X_1 则:
\begin{cases} X_1 = Z \\ X_2 = Y – Z \end{cases}, J = \begin{vmatrix} 0 & 1 \\ 1 & -1 \end{vmatrix}
\Rightarrow Y, Z 的联合密度为:
l(y, z) = f(z, y – z) |J| = f(z, y – z)
\Rightarrow Y 的 pdf 为:
l_Y(y) = \int_{-\infty}^\infty f(z, y – z) \, \mathrm{d} z = \int_{-\infty}^\infty f(y – z, z) \, \mathrm{d} z
注:
1. 若 X_1, X_2 独立,则:
l_Y(y) = \int_{-\infty}^\infty f_1(z) f_2(y – z) \, \mathrm{d} z
2. (X_1, X_2) 二元正态分布 \Rightarrow X_1 + X_2 \sim N(\mu_1 + \mu_2, \sigma_1^2 + \sigma_2^2 + 2 \rho \sigma_1 \sigma_2)。
No Comments